On June 15, the Methods Lab organized the workshop Introduction to Topic Modeling in collaboration with the research group Platform Algorithms and Digital Propaganda. The workshop aimed to provide participants with a comprehensive understanding of topic modeling – a machine-learning technique used to determine clusters of similar words (i.e., topics) within bodies of text. The event took place at the Weizenbaum Institute in a hybrid format, bringing together researchers from various institutions.
The workshop was conducted by Daniel Matter (TU Munich) who guided the participants through basic concepts and applications of this method. Through theory, demonstrations, and practical examples, participants gained insight into commonly used algorithms such as Latent Dirichlet Allocation (LDA) and BERT-based topic models. The workshop enabled participants to assess the advantages and drawbacks of each approach, equipping them with a foundation in topic modeling while, at the same time, providing plenty of new insights to those with prior expertise.
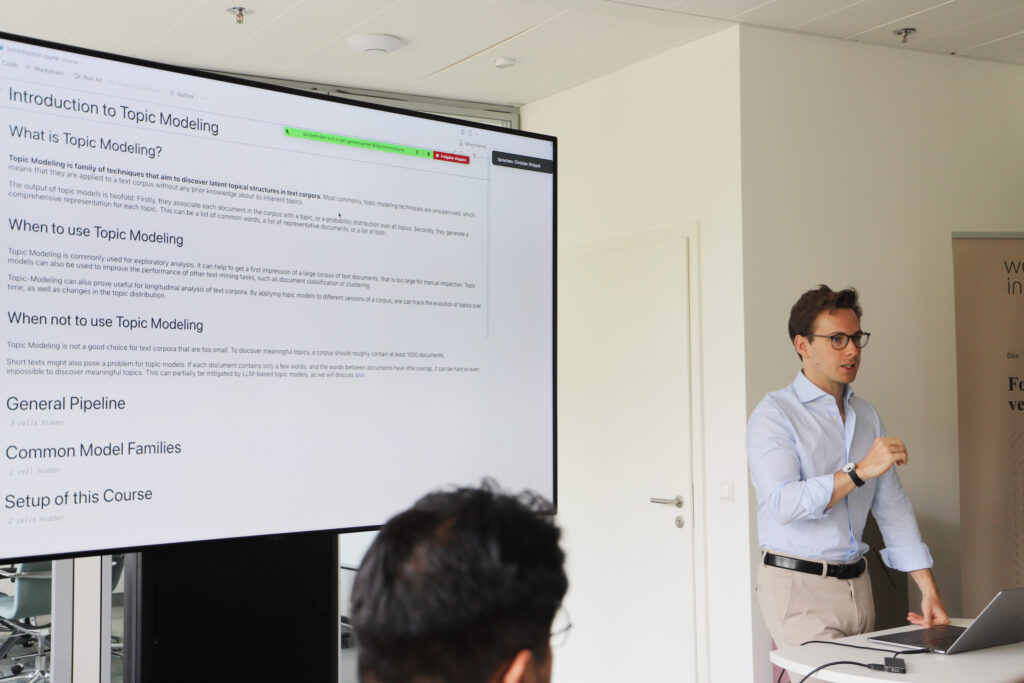
During the workshop, Daniel explained the distinction between LDA and BERTopic, two popular topic modeling strategies. LDA, or Latent Dirichlet Allocation, a commonly used method for topic modeling, operates as a generative model and treats each document as a mixture of topics. LDA aims to determine the topic and word distributions that maximize the probability of generating the documents in the corpus. With LDA, as opposed to BERTopic, the number of topics must be known beforehand.
BERTopic, on the other hand, belongs to the category of Embeddings-Based Topic Models (EBTM), which take a different approach. Unlike LDA, which treats words as distinct features, BERTopic incorporates semantic relationships between words. BERTopic follows a bottom-up approach, embedding documents in a semantic space and extracting topics from this transformed representation. Unlike LDA, which can be applied to short and long text corpora, BERTopic generally works better on shorter text, such as social media posts or news headlines.
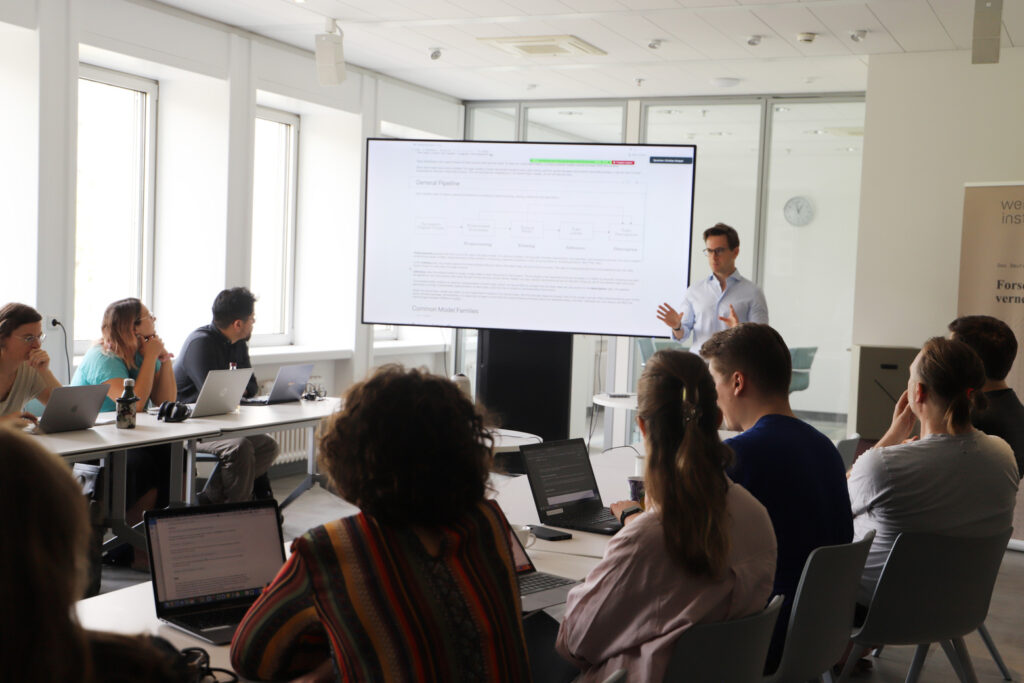
When deciding between BERTopic and LDA, it is essential to consider the specific requirements of the text analysis. BERTopic’s strength lies in its flexibility and ability to handle short texts effectively, while LDA is preferred when strong interpretability is needed.
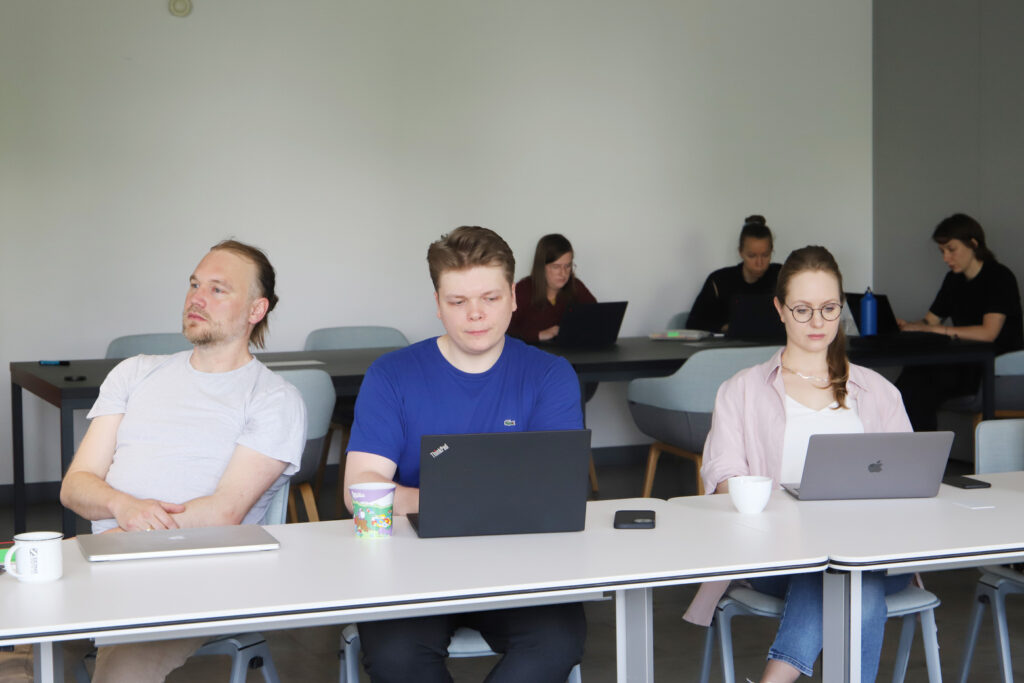
With this workshop, we at the Methods Lab hope to have provided our attendees with a solid understanding of topic modeling as a method. By exploring the concepts, applications, and advantages of each approach, these tools can be used to unlock hidden semantic structures within textual data, enabling researchers to employ them in various domains and facilitating tasks such as document clustering, information retrieval, and recommender systems.
A big thank you to Daniel for inducting us into the world of topic modeling and to all our participants!
Our next workshop, Whose Data is it Anyway? Ethical, Practical, and Methodological Challenges of Data Donation in Messenger Groups Research, will take place on August 30, 2023. See you there!