A third edition on the Introduction to Programming and Data Analysis with R workshop took place on March 12th and 13th, 2025. Roland Toth with the Methods Lab at the Weizenbaum Institute engaged almost 20 participants with essential methods of data analysis via comprehensive coverage of fundamental R programming concepts and techniques.
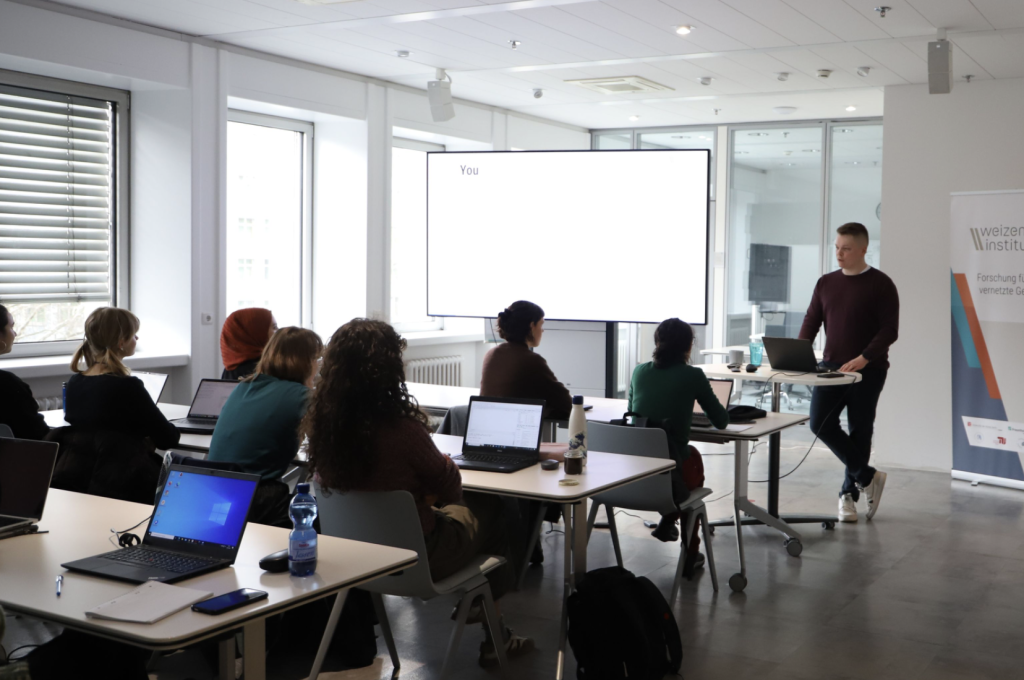
On the first day, Roland guided participants through the basics of R syntax and its integration with Markdown/Quarto in an interactive environment. This included the very basics of programming like functions, objects, and indexing, but also data-related practices like data wrangling, sanity checks, and simple statistical analyses. Among others, participants also gained insight on managing warnings and errors that might stunt the process of coding throughout projects.
On day two, after an introduction to data visualization techniques, participants put their learning into practice: They explored provided survey data and developed a research question, so they could prepare and statistically analyze the data accordingly in R. The result was a reproducible HTML report on the reasoning behind the research question, all data wrangling steps, an exploration of the data set, the analysis, and the results including an interpretation. Attendees also supported each other’s progress whenever possible, while Roland offered personalized guidance.
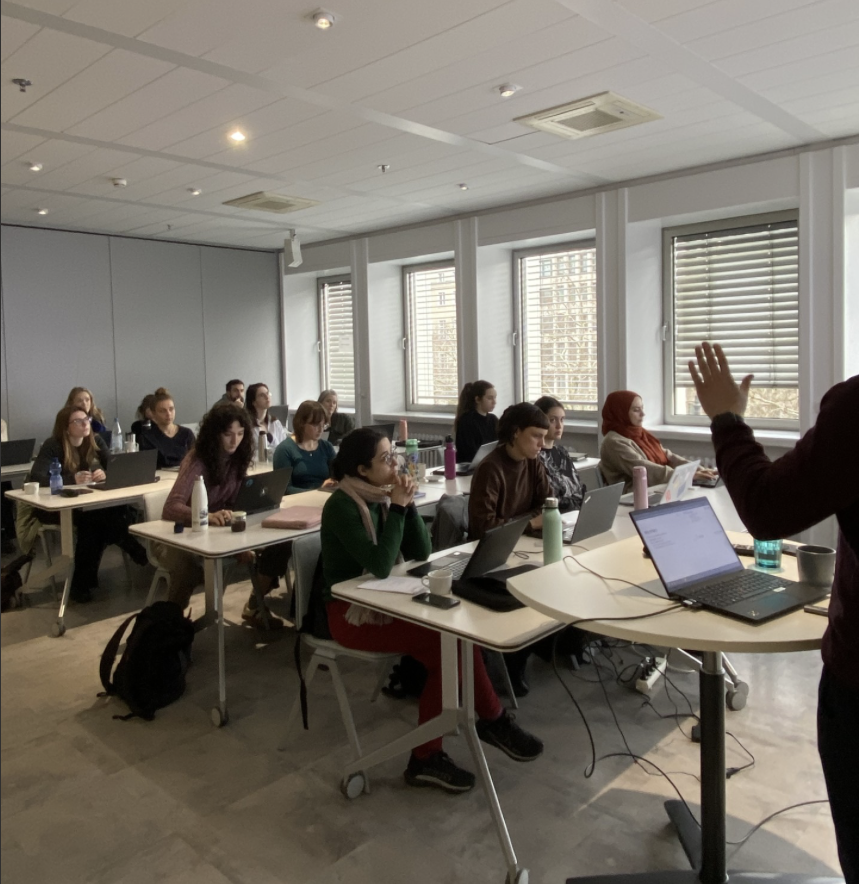
The workshop concluded with a thorough review of useful functions and packages in R. Throughout the event, participants were encouraged to ask questions freely and frequently, and they took the opportunity. The Methods Lab would like to give a great thanks to all guests for their attendance and lively participation!